Casino Crime Java
Even though there are various incidents of cheating the casino through the games provided in the building, more theft occurs than any other crime. Because a person uses a wallet or purse to contain winnings, someone steals these in excess to ensure enough has been taken to provide a good amount.
Data is the lifeblood of financial institutions and other organizations. It is used to run processes, manage financials, predict risk, prove compliance, target customers and influence decisions. In anti-money laundering (AML) and compliance, the data required to identify and combat financial crime is complex. It is also difficult to gather because data is often stored across a patchwork of legacy systems, new systems and siloed business-specific applications. Data quality can vary greatly. Working with unreliable, incomplete or inconsistent data makes it difficult to identify bad actors who pose a financial and reputational threat, which undermines an institution’s ability to efficiently manage risk across the enterprise.
The more complex and geographically diverse a financial institution is, the greater the threat. Institutions with very large customer databases and transaction volumes that span numerous distribution channels and counterparties face the greatest number of challenges and the most risk.
Apr 13, 2012 Broken down, violent crime jumped 28.57 per cent and property crime 15.98 per cent. Just north, traffic offences under the criminal code, such as impaired driving, shot up 41.51 per cent. Legalized gambling increases employment opportunities and, proponents argue, gives schools much-needed funds. But it also dramatically increases crime, bankruptcies, and addiction. Java online casinos offer both free play money and real money versions of their games. Once you sign up as a player, your account is a free account quite by default. This allows you to gamble without risking any cash, and it serves as the test drive of the casino software. Check out some additional advanced strategy as it relates to gambling and casino games in general: UK Slot Machine Betting Limit; Live Casino Roulette vs Standard Roulette; Make sure to visit Casinos Fellow, to choose the best deposit bonus casino to play.
The Slinging Arrows of Risk
Traditional AML defenses that rely primarily on static rules to identify questionable individuals and activities are coming up short. Despite continued investment in AML technology and processes, institutions seem unable to keep pace with external threats from drug cartels, corrupt public officials, terrorist organizations and other bad actors that have developed increasingly sophisticated tactics to avoid detection. These savvy criminals know how to play the game. They will often cloak their malicious activities by keeping within the defined set of rules. One example of how criminals fly under the radar is through smurfing. By limiting transactions to under $10,000, they avoid triggering a currency transaction report.
Internal threats—whether unintentional human error or intentional fraud—must also be considered when managing enterprise risk
Internal threats—whether unintentional human error or intentional fraud—must also be considered when managing enterprise risk. In addition to internal and external threats, emerging payment technologies and the digitalization of banking have introduced yet another set of risks to the AML landscape.
Cyber risk, social media monitoring and data management are all crucial considerations that have caught the attention of regulators, who have come to recognize that traditional, rules-based methodologies may not be optimal for certain typologies. Check-the-box compliance is not enough. Regulators expect banks to have defensive processes and systems in place to proactively seek out and catch perpetrators, whether external players or internal employees.
Big Data, Big Challenges
Know your customer (KYC) regulatory requirements have compelled institutions to collect increasing amounts of data on customers and their transactions. Static, rules-based systems are not designed to handle huge stores of unstructured, internet-scale data. As a result, they produce an enormous volume of false positive alerts. More data only produces more false positives when screening for sanctioned entities or money laundering.
Managing the deluge of false positive alerts is a major pain point for many institutions. Not only is the process inefficient and operationally expensive, but it complicates an institution’s ability to quickly and accurately identify risk. The knee-jerk reaction of “throwing more bodies” at the problem is not the answer. Adding resources just drives up the cost of compliance and increases the risk of human error.
Driving Change
The big data phenomena brought a proliferation of technology that can help meet the analytic and architecture challenges of AML, KYC and counter-terrorist financing. Data science, data analytics and other advanced technologies like artificial intelligence (AI) and machine learning offer a dynamic approach that is better suited to complex internet-scale data than static models. According to a report published by Celent, “AI-enabled solutions can not only automate significant parts of operations but also offer superior insights through advanced capabilities for analyzing structured and unstructured data.”1
These dynamic models focus on patterns rather than individual data points or transactions. They detect anomalies, making it easier to identify behavior that truly accounts for malicious activities. Dynamic models enable institutions to keep pace with changing requirements while also resolving the costly problem of reducing false positives.
Integrating non-traditional data sources into a data management program will improve the effectiveness of detection and ongoing due diligence. Non-traditional internal and external data sources can include documents, newsfeeds, images, video, social media, clickstream data and machine log data. Driving the growth of these variable data sources are an increase in client interactions and the digitalization of business processes.
While these new data sources offer a wealth of information for AML and compliance purposes, traditional structured query language-based analytic techniques may not be well suited for these non-traditional data sources because their pre-set schemas vary and change often. For this reason, an alternative approach for analyzing data uses programming languages such as Java, Python and R. These coding languages are often chosen for big data and analytical tools for several different reasons. For example, Python has become a popular choice for applications because it relies on the most cutting-edge techniques, such as AI, machine learning and natural language processing.
The ability to integrate non-traditional internal and external data sources enables institutions to go beyond basic analytics to identify risk more quickly and efficiently. When transaction data is enriched with client/legal entity data (including names, addresses and other identifiers), and publicly available OFAC lists, banks can track transactions to determine if they were completed by known high-risk individuals or non-cooperative jurisdictions. Going one step further, enriching this data with verbal and written communications information can help cast a wider net when looking at potential indicators.
Tip of the Iceberg
Fighting crime with big data and analytics still has a long way to go. Industry pioneers who wish to move beyond analytic technologies are looking toward cuttingedge solutions based on probability and inductive, heuristic logic that detects money laundering by replicating an analyst’s thought processes. This is the future state of advanced capabilities that institutions require to address comprehensive AML and compliance challenges in a dynamic environment. With the right investment in the right technology and data platforms, institutions can be confident that they have a clear view of risk across the enterprise.
In need of an AML Risk Assessment tool? ACAMS Risk Assessment® is the first AML risk assessment software of its kind to provide an automated means of measuring, understanding and explaining an institution’s money laundering risks. To request a free demonstration, visit http://www.acamsriskassessment.com/. You may also reach us directly via email at riskassessment@acams.org.
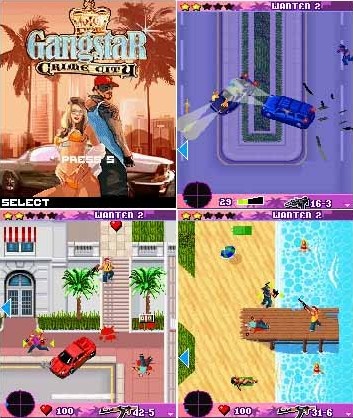
- Arin Ray and Neil Katkov, “Artificial Intelligence in KYC-AML: Enabling the Next Level of Operational Efficiency,” Celent, August 22, 2016, https://www.celent.com/insights/567701809
This copy is for your personal non-commercial use only. To order presentation-ready copies of Toronto Star content for distribution to colleagues, clients or customers, or inquire about permissions/licensing, please go to:www.TorontoStarReprints.com
Back in 2001, Toronto police weren’t painting a rosy picture of the neighbourhood surrounding Woodbine Racetrack.
Crime spiked, they said, after 1,752 electronic slot machines came on the scene in March 2000.
In the vicinity near the racetrack, overall criminal offences rose from 994 in 1999 to 1,267 the next year — a 27.46 per cent increase, according to a 2001 police report. Broken down, violent crime jumped 28.57 per cent and property crime 15.98 per cent. Just north, traffic offences under the criminal code, such as impaired driving, shot up 41.51 per cent.
The data, while somewhat dated, shows gaming can cause ancillary problems for a neighbourhood — at least initially.
While police said Friday that more recent statistics for the area around the slots were not immediately available, one officer on the beat for the last 10 years said he’s seen a steady decrease in crime.
It’s an issue GTA residents will have to ponder when deciding whether they want a casino in their midst.
The province is reopening the issue of casinos as part of a reorganization of gaming, arguing it could add $1.3 billion to public coffers. But it has left any final say up to municipalities.
This week, Toronto City Council postponed deciding whether to hold a referendum, instead voting to send the issue to an executive committee. In 1997, residents of all six of the former municipalities that now make up Toronto rejected allowing a casino.
The 2001 report was prepared for the Toronto Police Services Board for the purpose of asking for 18 additional officers to deal with the onslaught. While police were careful to say the slots were not solely responsible for increases in crime, the report said their introduction was a “contributing factor.”
One indicator of the effects of gaming on the neighbourhood was that more pawn shops and second-hand stores were popping up, it said.
Toronto police Supt. Ron Taverner said crime rates around the racetrack these days are relatively low compared with other areas of the city. “From a policing perspective, I don’t see any issues at all,” he said, adding that fears of increased prostitution, loan sharking and even traffic flow and parking problems didn’t pan out.
On Thursday, Police Chief Bill Blair echoed the sentiment, saying, “I certainly don’t perceive it’s been a significant public safety issue for us. It’s a well-organized, well-managed business, and they work very closely with the police.”
At the time the report was prepared, Julian Fantino, now the Conservative MP for Vaughan, was Toronto’s chief of police.
Casino Crime Javascript
Retired Queen’s University law professor Stanley Sadinsky isn’t convinced that crime automatically comes with casinos. In Windsor, Ont. — home to one commercial casino — crime rates actually went down, he said.
Sadinsky added that he thinks police departments have also seen the introduction of casinos as an occasion to increase their manpower. “There was this worry that there would be a problem, and monies were suddenly available to municipalities. If I were the chief of police I’d make the case for more resources as well.”
Casino Crime Java Download
Copyright owned or licensed by Toronto Star Newspapers Limited. All rights reserved. Republication or distribution of this content is expressly prohibited without the prior written consent of Toronto Star Newspapers Limited and/or its licensors. To order copies of Toronto Star articles, please go to:www.TorontoStarReprints.com